
Novelty Detection
Novelty detection refers to the identification of events that do not conform to expected behavior. Standard classification settings are infeasible for the goal since the nature of novel examples cannot be known a priori. For such reason, we tackle the problem in a semi-supervised learning setting. We are interested in the formalization and assessment of novel models capable of learning the distribution of regular events and deem as novel the ones which are less explicable in a probabilistic sense.
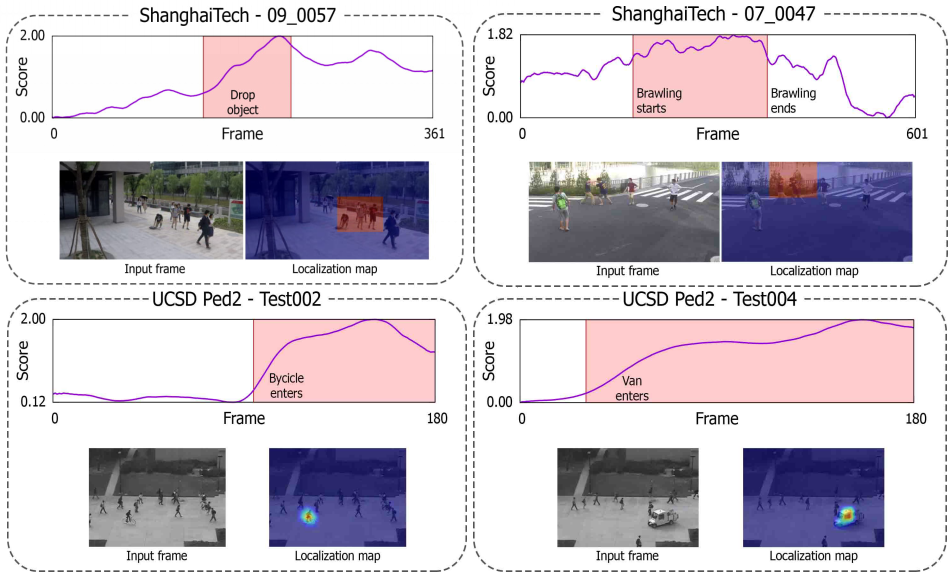
Novelty detection belongs to the family of anomaly detection problems. As such, it has a long history in computer vision and has significant relevance in diverse applications in industry (from video surveillance to defect/fault detection) and in research (from active learning to exploration in reinforcement learning). Somewhat informally, it can be defined as the identification of data which exhibit significantly different traits from a collection of typical examples. A more precise formulation describes the task as the problem of finding a scoring function in a binary classification setting, under the constraint that the training dataset contains examples from one class only (the normal one).
Indeed, novel examples are sporadic in real-world settings, and it is costly to acquire them in a sufficient number. Moreover, the cardinality of all possible novel instances is often intractable.
The semi-supervised nature of the problem makes it extremely challenging.
Our efforts in this field led to the development of a deep neural network, leveraging end-to-end training to learn features that characterize most normal examples: novelties are identified according to such traits.
Specifically, we introduce:
- a model based on a deep autoencoder, as well as a regularized objective suitable for novelty detection settings. Such regularizer is based on an additional module responsible for the parametric estimation of the density function of bottleneck vectors;
- the use of an autoregressive procedure, ensuring proper density estimates;
- a theoretical intuition advocating for the suitability of the proposed regularization in novelty detection settings, based on the informative content of novelty (high information) against normality (low information).
Extensive experiments show state-of-the-art performances both in controlled one-class experiments and in large-scale video benchmarks. Notably, such performances were achieved without any domain-related assumption, making our proposal very general and applicable in disparate settings.
As a future step, we'd like to extend our work to non-stationary settings, in which the distribution of normal examples shifts in time and the model has to adapt accordingly.