
Bias-Free Artificial Intelligence methods for automated visual Recognition: detecting human prejudice in visual annotations and mitigating its effects on models’ learning (B-FAIR)
Modern AI algorithms are capable of reproducing human skills and can even outperform people in several tasks if they have access to large enough training data. Such a technical leap has raised the paradox that algorithms, being nourished with human annotated data, tend to absorb the bias hidden in the data itself. As a consequence, AI systems may exhibit sexist or racist behavior simply because this attitude is present in the people that produce their training data. Stereotypes on race/ethnicity, in particular, may introduce significant bias in AI learning process, especially with the recent escalation of racist behaviour intensified by the migrant crisis that is challenging EU countries and Italy.
B-FAIR aims at analyzing, understanding and counteracting the effect of racial prejudice bias in visual recognition systems. First, it will stimulate the hidden prejudice bias in human annotators by presenting them large-scale visual datasets featuring racial diversity generated with modern AI techniques. Then, it will develop algorithmic countermeasures to AI systems’ racist behaviors. B-FAIR will pilot its novel approach in two scenarios: cyberbullying prevention and detection of dangerous situations in video surveillance.
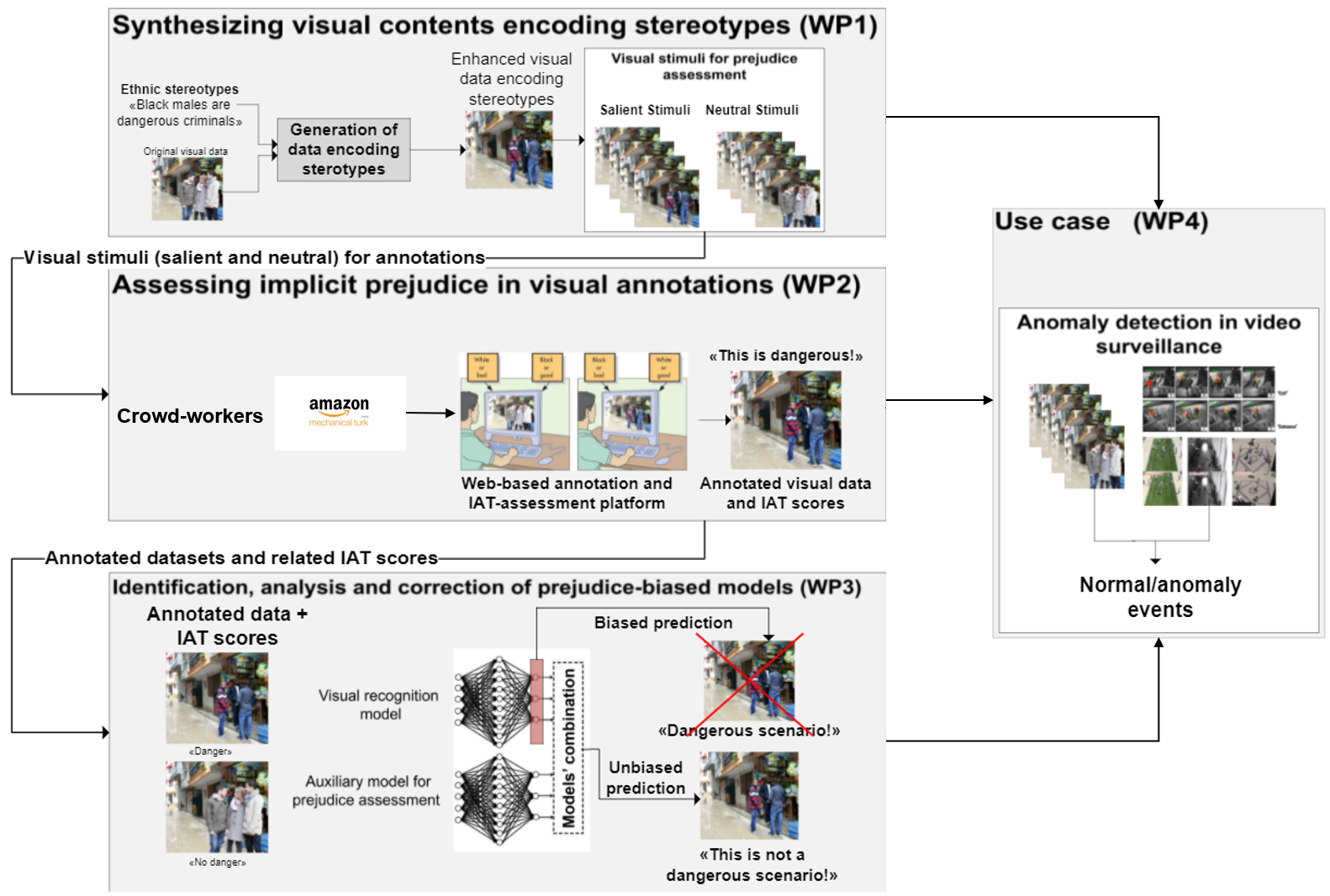
Here we cover the research areas that are the main focus of B-FAIR.
UNCOVERING ANNOTATION BIAS IN VISUAL RECOGNITION: The problem that AI models trained on visual datasets may not generalize to real-world scenarios, or worse, may perpetuate prejudice and stereotypes (i.e. related to gender, race, etc) inherently present into human annotations has been studied for many years [1,2]. Lately, this issue has become more evident, as training deep networks requires large scale datasets with annotations acquired with crowdsourcing. For instance, [3] analyzed image descriptions generated by crowdworkers in the Flickr30K database, discovering patterns of sexist and racist bias in the associated image captioning systems.
While the dataset bias issue has been evident for quite some time, its technical implications have been much less studied. For instance, a related problem is how to automatically analyze a trained model to understand if it is biased. This problem is especially challenging for deep networks, as their lack of decomposability into human-understandable parts makes them hard to interpret. Recently, the research community has made significant progress, developing Explainable AI methods [4]. Specific efforts have been made in computer vision, e.g., designing deep networks which not only output predictions but also the associated textual or visual explanations [5,6]. Other studies have adopted these methods to analyze models affected by prejudice-induced bias [7]. However, these methods have many limitations, e.g. they require human feedback for semantic interpretation, or are limited to specific deep architectures.
IMPROVING DEEP MODELS BY COUNTERACTING BIAS: Devising technical solutions to neutralize prejudice-induced bias in annotations is important not only for improving the generalization performance of AI models, but also for fair and ethical outcomes as more algorithmic decisions are made in society. Recently, the AI community has devoted particular attention to address this task. A simple strategy to remove or at least alleviate the effect of bias is by manually curating and assessing the quality of training data. This strategy is currently adopted by many companies [8]. In particular, significant efforts have been made for creating large image collections for training visual recognition systems, e.g. in order to balance face appearance with respect to race and gender [9,10]. For instance, in 2015 IARPA released the IJB-A dataset as the most geographically diverse set of collected faces [9]. However, collecting large and diverse datasets alone cannot suffice to learn fair and trustworthy AI models. Therefore, recently, several algorithmic solutions to automatically counteract bias have been proposed [11,12,13]. However, the vast majority of these works do not focus on methodologies for explicitly dealing with visual data, despite biased visual systems can have serious societal implications.
CONDITIONAL VIDEO SYNTHESIS FOR IMPLICIT PREJUDICE ASSESSMENT: Generative Adversarial Networks (GANs) [14] have revolutionized visual data synthesis, with recent style-based generative models boasting some of the most realistic synthetic imagery to date as well as arbitrary modification of visual attributes, e.g., changing facial attributes or style [15, 16]. Significant progresses have been made also in developing AI models for generating extremely realistic but synthetic videos (e.g. deepfakes) [17, 18] or for conditional image/video generation (i.e. that either create artificial images or videos starting from text descriptions) [19]. However, current techniques for manipulating visual input may not suffice for supporting the assessment of implicit prejudice as we plan in B-FAIR, especially when dealing with complex video sequences where multi-object behaviour and motion information must be taken into account.
QUANTITATIVE ASSESSMENT OF IMPLICIT PREJUDICE: Nowadays the outward expression of prejudice is not socially acceptable and has been replaced by subtle or implicit prejudice, which is assessed with implicit measures. These are experimental paradigms that infer mental associations between concepts (e.g. the association between an ethnic minority and a negative evaluation) from participants’ performance in categorization tasks. The most widely used implicit measure is the Implicit Association Test (IAT) [20]. Considering it within a social perception task requires annotating minority and majority visual stimuli (i.e. salient/stereotypical and neutral/non-stereotypical). However, collecting salient visual contents is not trivial and often requires labor-intensive activity by psychologists, which calls for computer-based methods to automate the process. A few attempts of exploiting AI for IAT (and implicit prejudice) assessment have been proposed in the literature [21,22], but to date there are no methods supporting the automatic generation/collection of visual stimuli as we plan in B-FAIR.
Project Info
